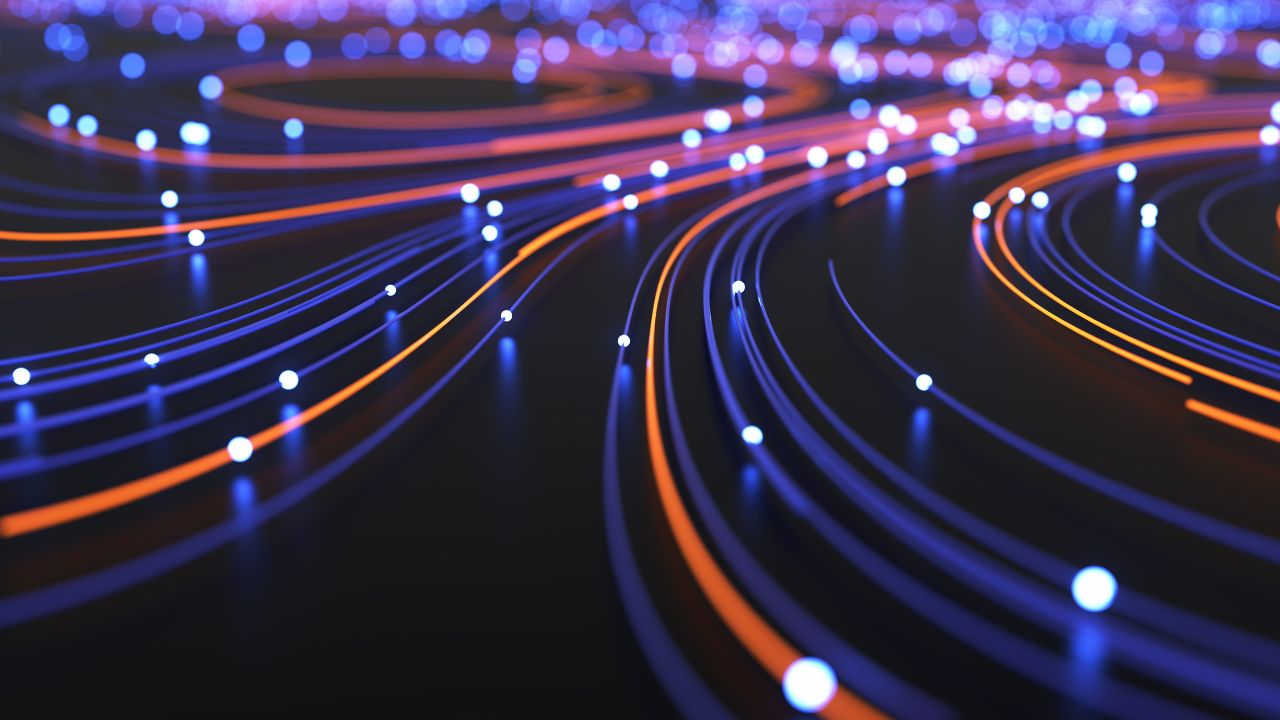
As data becomes more democratised, new players are entering the commodities space, ramping up the competition. Today, success hinges less on having access to data and more on how you use it. That’s where a strong technical team makes all the difference. In this series, we’re speaking with tech leaders in the commodities industry to gain their insights on how to thrive in this data-driven landscape.
We recently had the pleasure of speaking with Anton Bakharevski, a seasoned leader in the commodities industry with extensive experience at major companies such as Shell, Noble Group, Genscape, Goldman Sachs, and Barclays Capital. Over the years, he has held senior positions, including CTO, Head of Development, VP of Engineering, and Chief Systems Architect. He has overseen technology teams that provide crucial systems development and support to various commodity trading organisations, from physical commodities to highly sophisticated exotic derivatives trading.
What’s the biggest challenge related to becoming a data-centric organisation?
A key challenge is to democratise access to data within your company. Most organisations hold vast amounts of proprietary information, but it’s dispersed across multiple divisions and technologies. Given the sheer amount and diversity of the data, the starting point should be to capture the data and make it available to the rest of the organisation.
Companies undergoing data-centric transformations have been trying to achieve this successfully for decades. In the 90s, people talked about concepts like data warehouses, OLAP (Online Analytical Processing), and OLTP (Online Transaction Processing). These terms represented early efforts to manage and analyse data. Over the years, this has evolved into a new set of technologies and approaches. Today, people talk about data lakes, data-driven organisations, data mesh, and data virtualisation. These are all evolutions of earlier attempts, reflecting advancements over the past 30 years. Despite these developments, effectively managing and integrating data remains challenging. It’s both a question of the data source – whether the input data is available and something you can process – and also whether you have a system that allows you to store and process that data.
What’s the biggest mistake commodities companies make with technology?
I feel many companies are not treating technology as a vital component of their competitiveness and are consequently not giving technologists a seat at the leadership table. There is a chasm between the business and technology, where leadership does not understand the technology, and technologists struggle to explain the benefits to gain buy-in. This stifles decision-making and innovation, resulting in a fragmented technology footprint and half-finished initiatives.
Many COOs and CFOs, who often lack technology acumen, struggle to understand the technology investment dynamic and how ROI is realised. Yet, they are often appointed to oversee company technology. Sure, while they can recognise the value a front office developer brings by seeing the reports and new functionality created, the use of enterprise technology and a standard data mesh remains an abstract concept to them.
I once worked for a major investment bank that did things the right way. Their technology teams are led by technologists who have a history of coding. As a result, it is one of the few investment banks with a single unified set of technology across the entire organisation for handling all types of data. Even with a leaner technology team than other firms, they can support all their diverse businesses (not only commodities) while benefiting from a faster rate of delivery.
When hiring data scientists, how important is commodities industry knowledge?
Technologists often have to make assumptions about areas outside their expertise and proceed until proven right or wrong. This applies to both technical and functional domains. The better they know the industry and the more they can make connections and draw parallels with other success stories, the higher the chance their assumptions will be correct and projects will deliver significant value. That’s why subject matter expertise does matter, ultimately it helps deliver higher value to the business.
What advice would you give when hiring data scientists?
Cleaning and organising data is one of the most time-consuming parts of data science, so it’s important to manage your candidates’ expectations. One of the interview questions I ask data scientists is what percentage of their time they spend on data management and data engineering versus actual data science. I have seen very few cases when this ratio was better than 80:20!
Today, most data scientists still spend a significant, if not the majority of their time, identifying the data they need, acquiring it, assisting data engineers, and evolving data models. Only then can they begin their core work. The current challenge is to shorten the cycle between data engineering tasks and data science so that data scientists can focus more on their primary work. Only then the 80:20 ratio will improve.
How do you see AI impacting commodities?
AI is developing at a fascinating pace, creating more and more opportunities to leverage it. From filling out trade blotters to identifying arbitrage opportunities, AI can automate many tasks or act as a knowledgeable assistant to speed up routine work. These opportunities will continue to emerge as companies succeed in democratising data currently locked away in various systems and in improving data timeliness and quality.
Looking for more insights?
Get exclusive insights from industry leaders, stay up-to-date with the latest news, and explore the cutting-edge tech shaping the sector by subscribing to our newsletter, Commodities Tech Insider. Interested in being featured in a future spotlight? Reach out to [email protected].
Modal Title
About the authors
Read more blogs
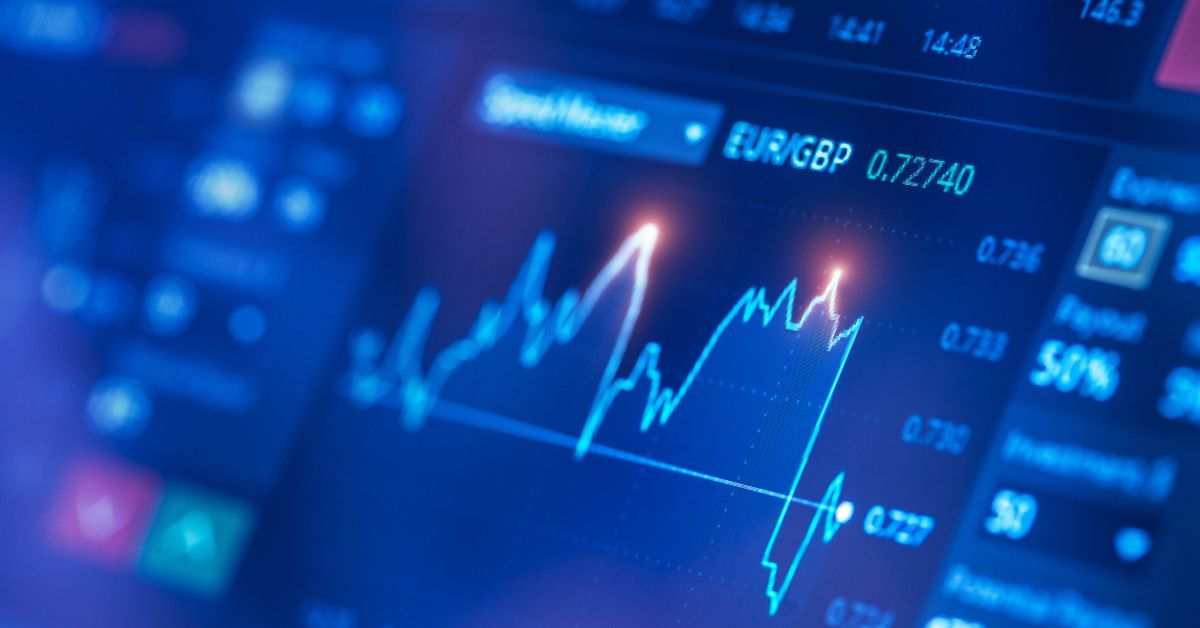
The most successful commodities trading firms don’t rely solely on intuition: they harness advanced data capabilities and invest heavily in their technology teams. Take Citadel, for example. When Enron, a major energy trading firm, filed...
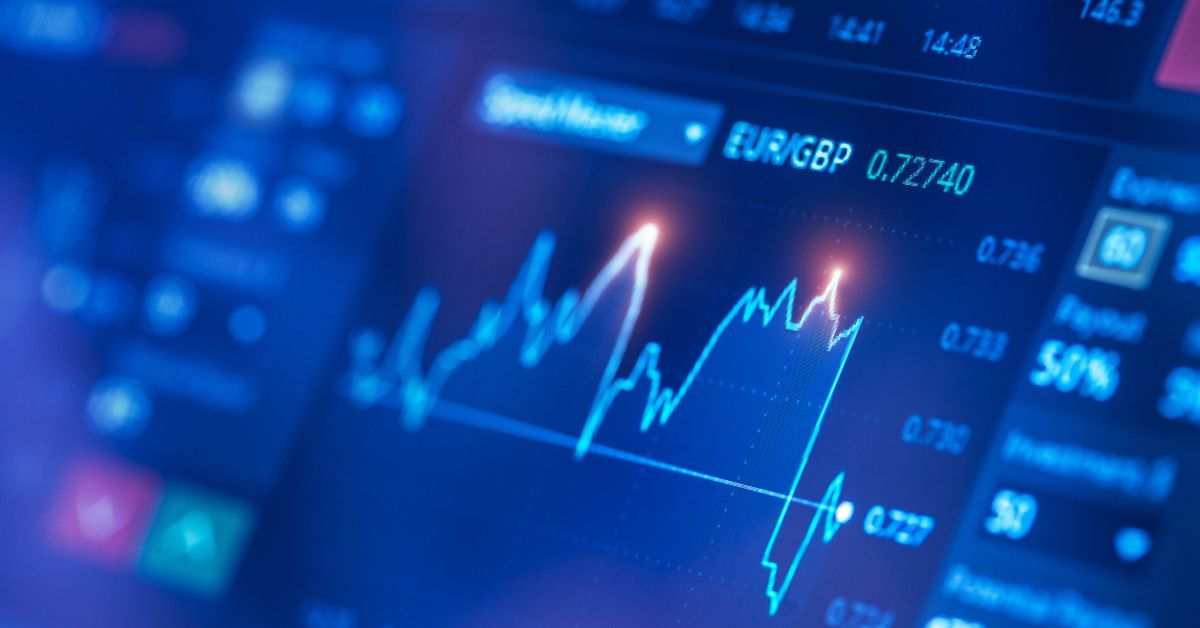
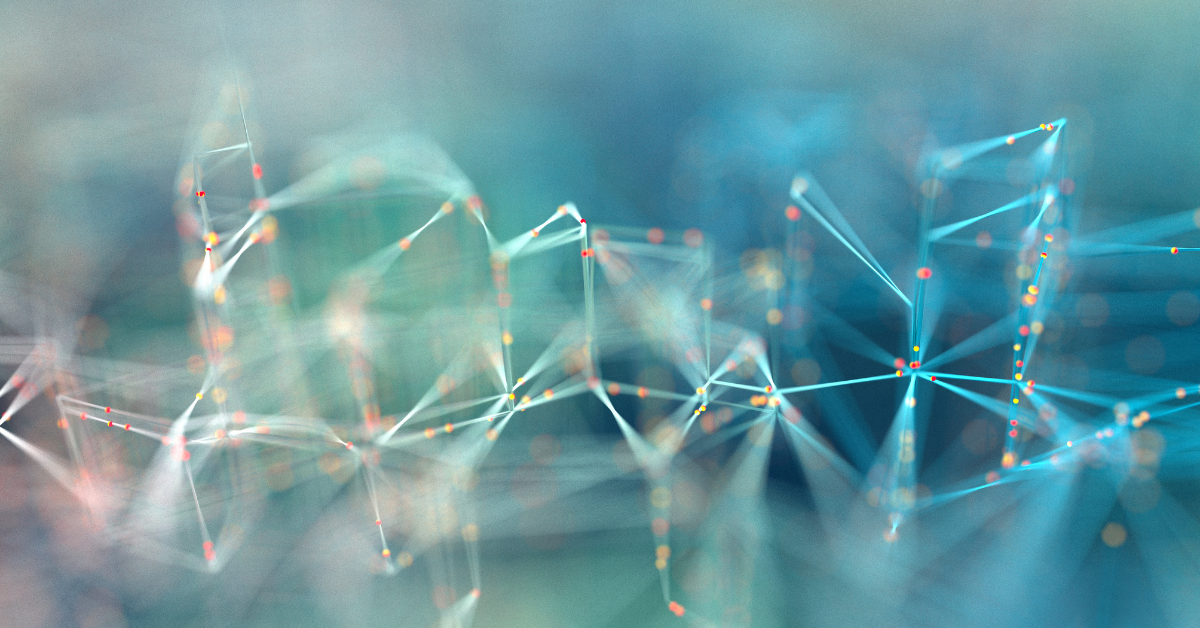
Today’s commodities markets are more unpredictable than ever. Geopolitical tensions, rising inflation, and the energy transition are all contributing to the volatility. To manage risks and find new opportunities in this competitive environment, industry players...
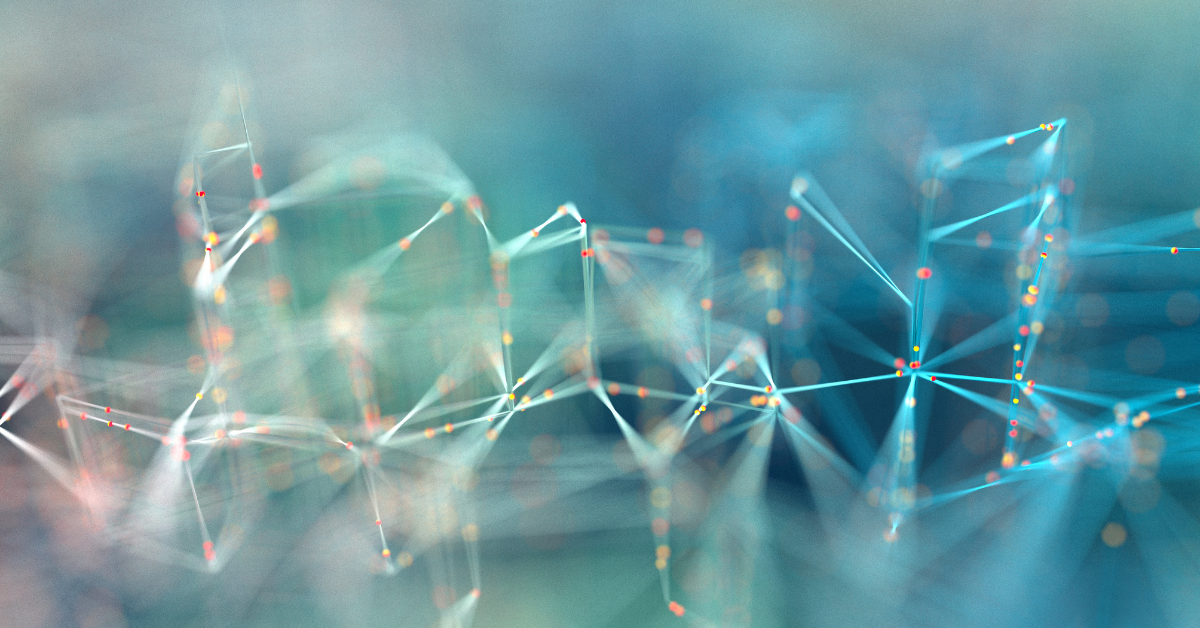
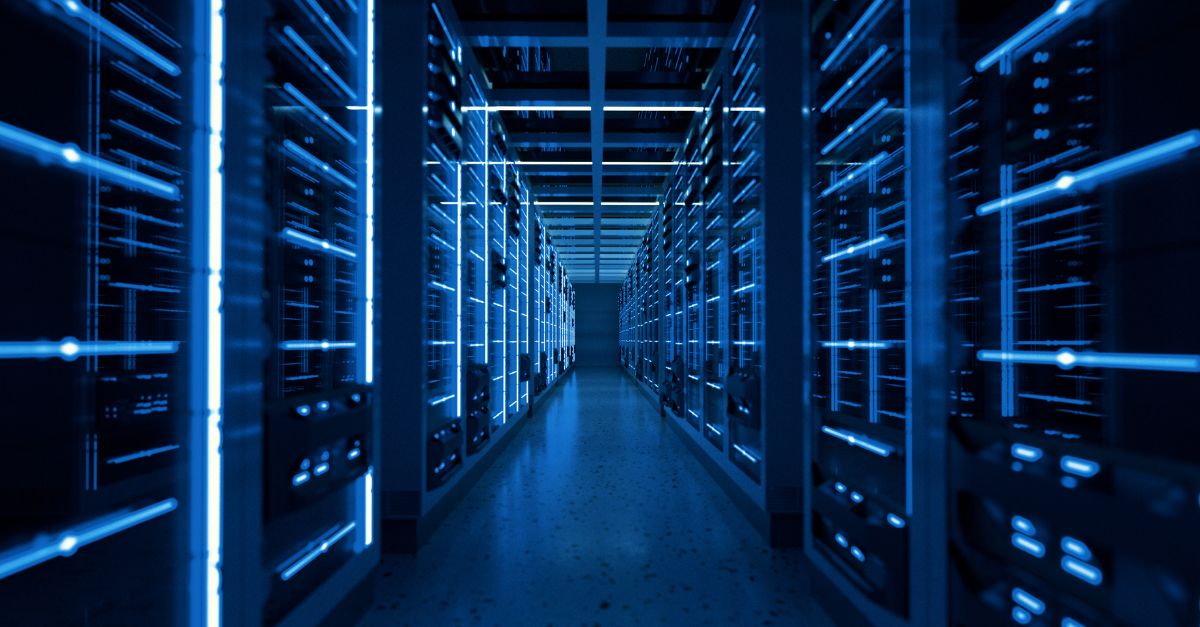
As data becomes more democratised, new players are entering the commodities space, ramping up the competition. Today, success hinges less on having access to data and more on how you use it. That’s where a...
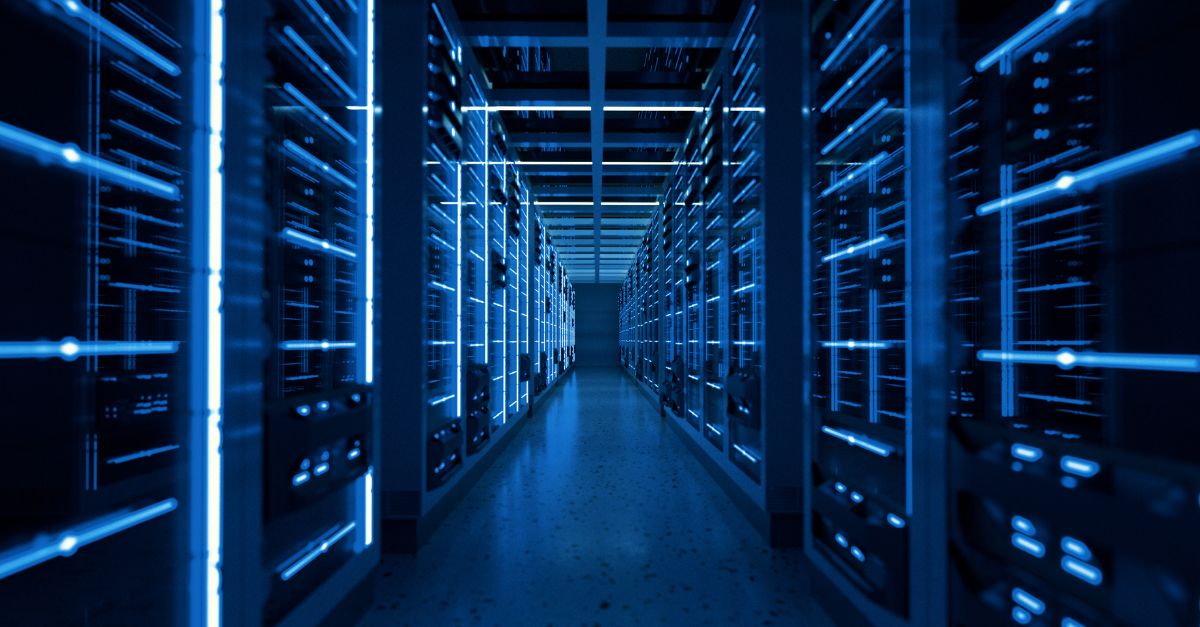