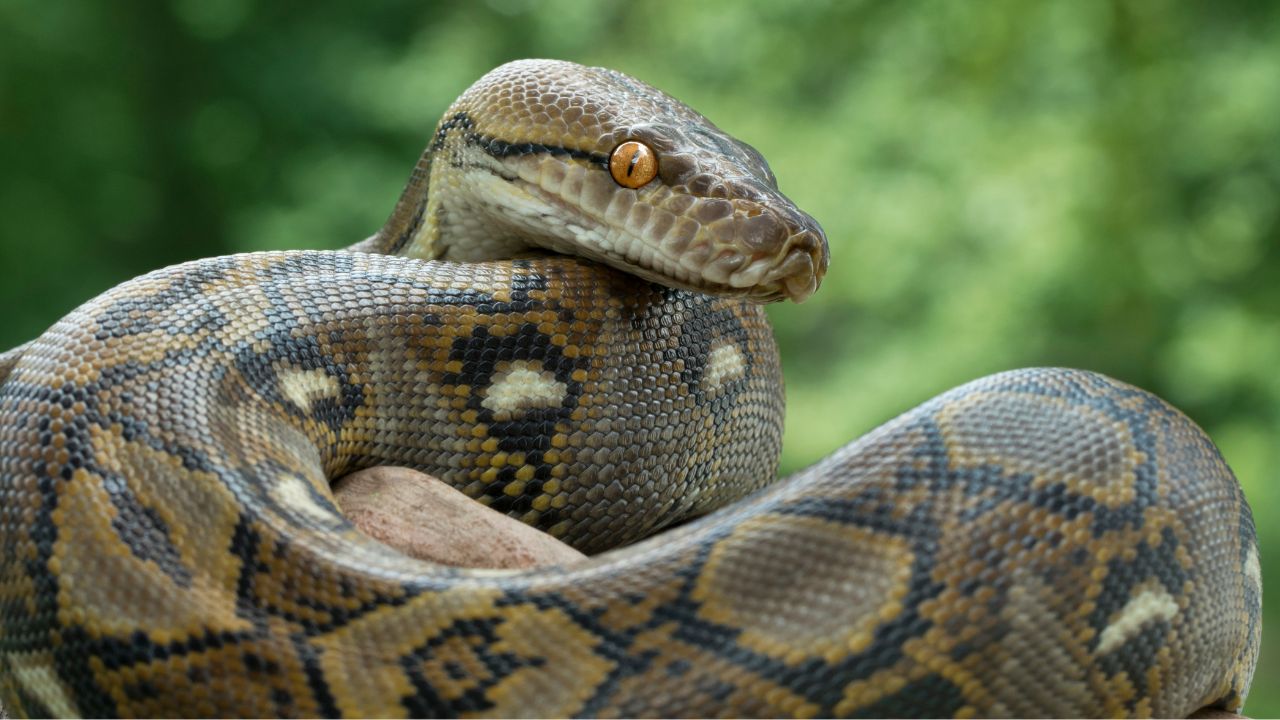
Python is a programming language that’s rapidly becoming the go-to tool for commodities traders, analysts, and risk managers alike.
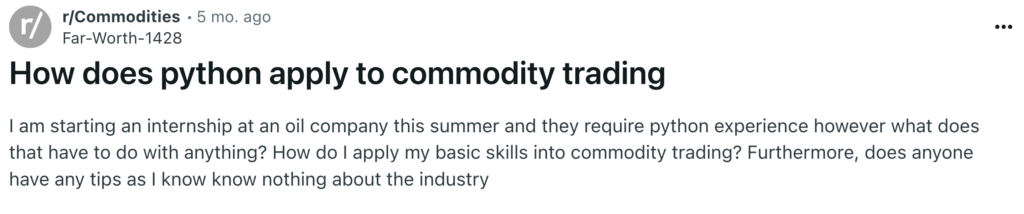
It’s a curious paradox. On one hand, there’s the stereotype of the seasoned trader, relying on gut instinct and years of experience. On the other, there’s the data-driven analyst, armed with Python, diving deep into complex datasets.
And the truth lies somewhere in between.
Data is the new commodity. From shipment movements to weather patterns, geopolitical events to consumer trends, information is pouring in from every angle.
Traditionally, analysts and traders relied on spreadsheets and statistical packages to make sense of this data. However, as data volumes exploded, the limitations of these tools became apparent, with manually processing all these data sources like trying to drink from a fire hose.
Why Python?
Python, with its powerful libraries like Pandas, NumPy, and Matplotlib, provides traders with essential tools to manage vast datasets, identify patterns, and uncover hidden opportunities. At the same time, its statistical capabilities help traders assess the probability of future market movements, calculate risk metrics, and build predictive models.
Python powers the full ‘loop’ in a trading cycle where data engineers build the data foundation, data analysts extract insights, and traders make the informed decision behind the data-driven trade. Beyond data analysis, Python is also being used to build sophisticated models. These can simulate market behaviour, assess risk, and optimise trading strategies. The ability to quantify and test different scenarios is invaluable in a market as volatile as commodities.
What’s behind the sudden surge in Python’s popularity?
Part of it is the language’s simplicity and versatility. It’s relatively easy to learn but still powerful enough to tackle complex problems. The growing community of Python users in finance, who share code, libraries, and best practices, also plays a big role. Plus, the steady stream of Python-skilled graduates from educational institutions is making it easier for the language to gain traction in commodities trading. Python is now one of the favoured programming languages in universities, ranked highly by UK educators for its ‘pedagogical benefits, meaning it’s easier to teach and learn. And on the boot camp front, the market size reached US$ 597.8 Million in 2023. Looking forward, IMARC Group expects the market to reach US$ 1,493.7 Million by 2032, exhibiting a growth rate (CAGR) of 10.71% during 2024-2032 and in turn, even more seasoned Python developers.
The future of commodities trading is increasingly driven by data, and Python is emerging as the premier tool to navigate this evolving landscape. Its flexibility, powerful libraries, and growing community make it the ideal language for leveraging data to drive informed trading decisions and develop sophisticated strategies.
Looking for more insights?
Get exclusive insights from industry leaders, stay up-to-date with the latest news, and explore the cutting-edge tech shaping the sector by subscribing to our newsletter, Commodities Tech Insider.
Modal Title
About the authors
Read more blogs
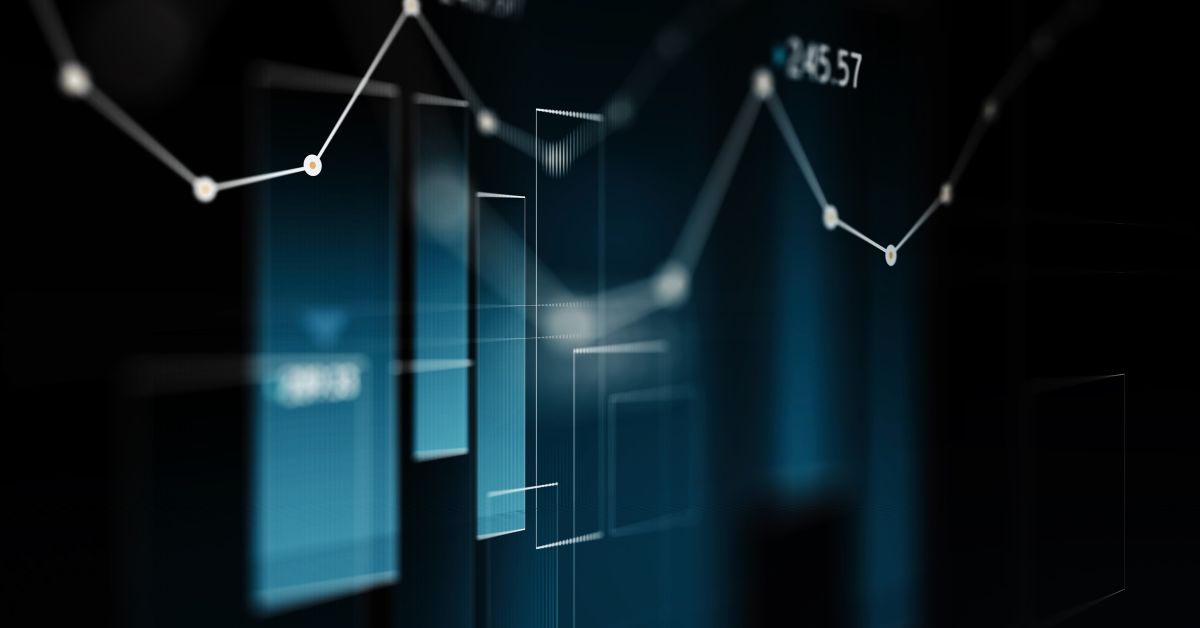
Data is the backbone of decision-making in today’s organisations, but its true value can only be unlocked through a clear, well-structured journey. This journey starts with the collection of raw data from diverse sources, followed...
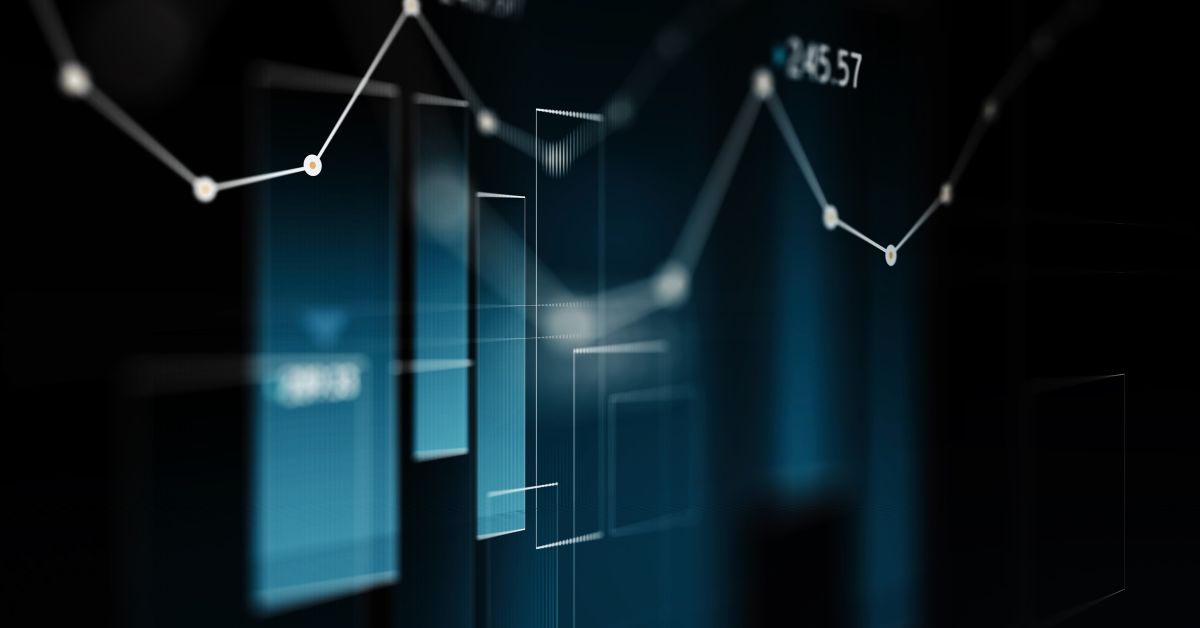
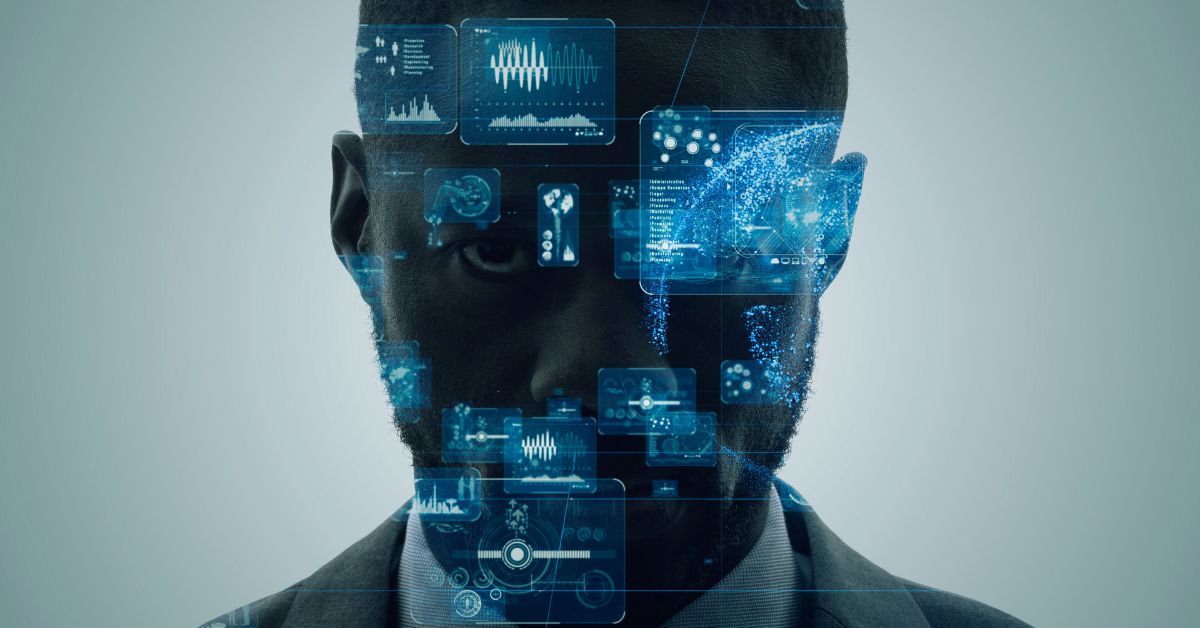
Trust is the invisible foundation that keeps our lives moving. When you step into a taxi, you trust the driver to get you to your destination safely. When you visit a doctor, you trust their...
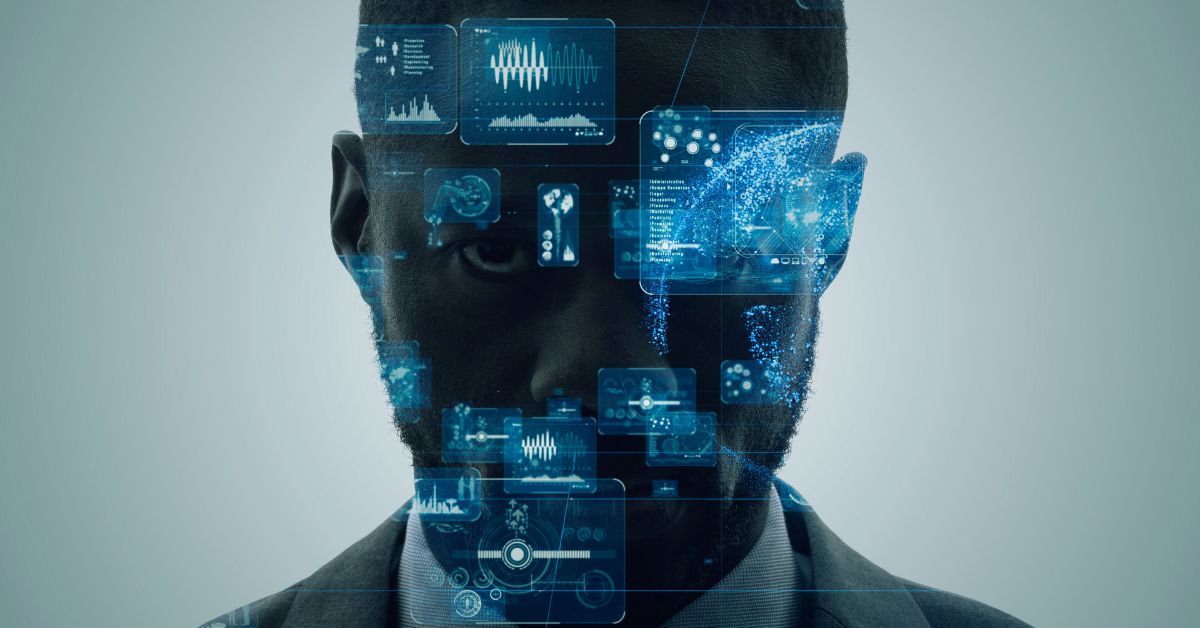

Today’s commodities markets are more unpredictable than ever. Geopolitical tensions, rising inflation, and the energy transition contribute to volatility, attracting new entrants such as banks and tech-focused trading players. To manage risk and find opportunities...
