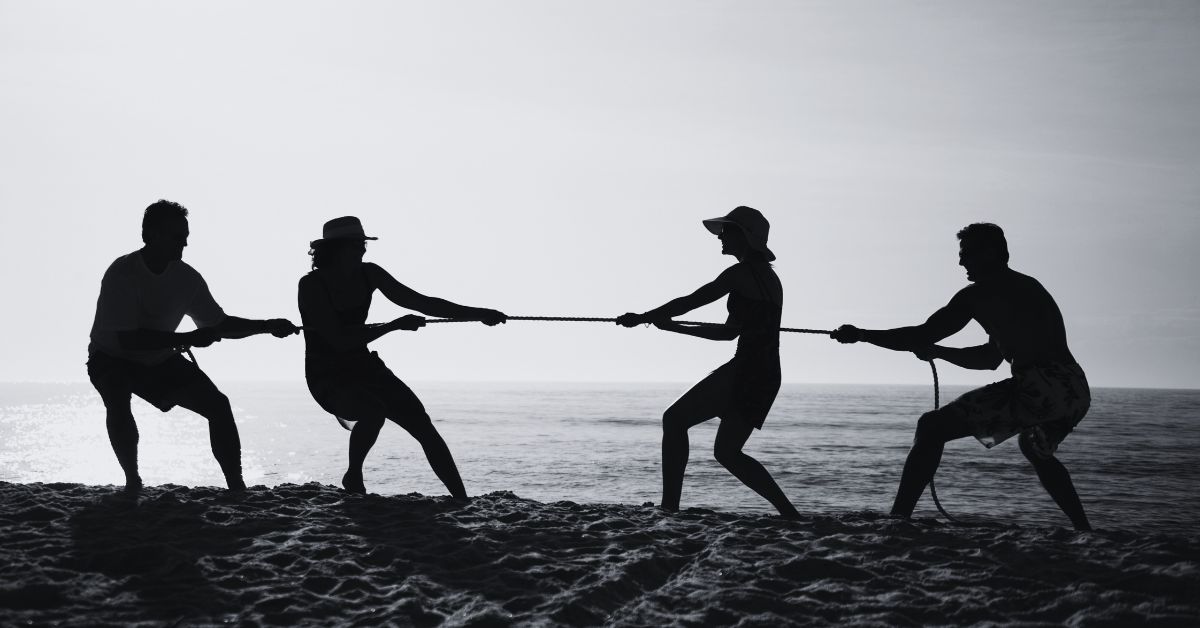
Why do humans feel the need to ask why?
It’s a question that’s shaped our existence for millennia. From the dawn of civilisation to the present day, our need for understanding has driven us to explore, learn, and challenge the world around us. This instinct to seek answers is not merely curiosity – it is central to what it means to be human.
But, when it comes to Artificial Intelligence (AI) the most cutting-edge models are also the most opaque. They make connections across datasets at a scale no human could replicate, delivering predictions and insights that can revolutionise trading. Yet, explaining these insights often reduces them to oversimplified narratives—a human filter on something that doesn’t fit neatly into human language.
In trading, where profit margins can be razor-thin, even a marginally better model can be the difference between success and failure. Yet, behind the scenes, there’s a constant tug-of-war. Trying to make an AI model more explainable, by understanding why it made a decision, often means sacrificing accuracy, and vice versa.
Which is more important? Well, that’s up for debate.
Where every split-second decision could mean millions gained or lost, does it really matter why an AI model makes a call if you have already decided to deploy AI? Jack Nugent, Director at Tradavex Ltd, likens the push for explainable AI to “choosing a wooden cart over a Ferrari: the cart is easier to understand but you’d never take it into a race.” His argument is simple: if humans have already decided to use AI, why compromise its accuracy for the sake of a narrative?
“Simplifying AI models to make them explainable often sacrifices accuracy. It’s like choosing between a wooden cart and a Ferrari: the cart is easier to understand but you’d never take it into a race.”
Jack Nugent, Director at Tradavex
In most cases, you also have to ask what value an explanation provides. Patrick Bangert, VP and Chief of AI at Oxy, says, “Many so-called explanations generated by AI systems are not helpful to their users. Often, it is more helpful to simply know how accurate the original prediction was.”
On the other hand, explainability scratches a very human itch—the need to understand and the need to ask why. According to McKinsey, companies that see significant returns from AI, attributing at least 20% of their profits to it, are more likely to follow best practices that promote explainability, leading to greater trust and better outcomes. As Tristan Fletcher, co-founder of ChAI, shares, explainability is important for getting buy-in. For him, “At ChAI, we knew that when predicting the direction of a commodity’s price, it’s essential for our clients to understand why those predictions have been made—not least because they might need to explain those decisions to their stakeholders.” If addressing this fundamental instinct is key to buy-in, doesn’t that make it important and not something that can be overlooked?
Ultimately, though, this is all about human judgment calls rather than algorithms. As Jack Nugent puts it: “It’s always good to ask why! It’s not possible to blindly follow AI outputs in commodity trading anyway. Humans have already decided what training data to use, what to do with the outputs, etc. So the question shouldn’t be, ‘Why did the AI model give us that output?’ Instead, it should be, ‘How do we decide what to do with the AI output?’ or ‘Why should we use AI in the first place?’ These are naturally human questions asked in a human way that need a human response. AI explainability cannot satisfy that.”
Given that this tug-of-war is set to continue, it’s still up for debate which approach trading teams should take:
Should you explain to your stakeholders why the AI model arrived at its output as justification for your actions?
Or should you need to explain why you chose to act based on the AI model’s output?
Over to you.
Looking for more insights?
Get exclusive insights from industry leaders, stay up-to-date with the latest news, and explore the cutting-edge tech shaping the sector by subscribing to our newsletter, Commodities Tech Insider.
Modal Title
About the authors
Read more blogs

The commodities industry has traditionally been male-dominated, especially at the leadership level. In 2018, Bloomberg revealed that women held less than 5% of senior management roles at top commodities traders. In the energy sector, S&P...

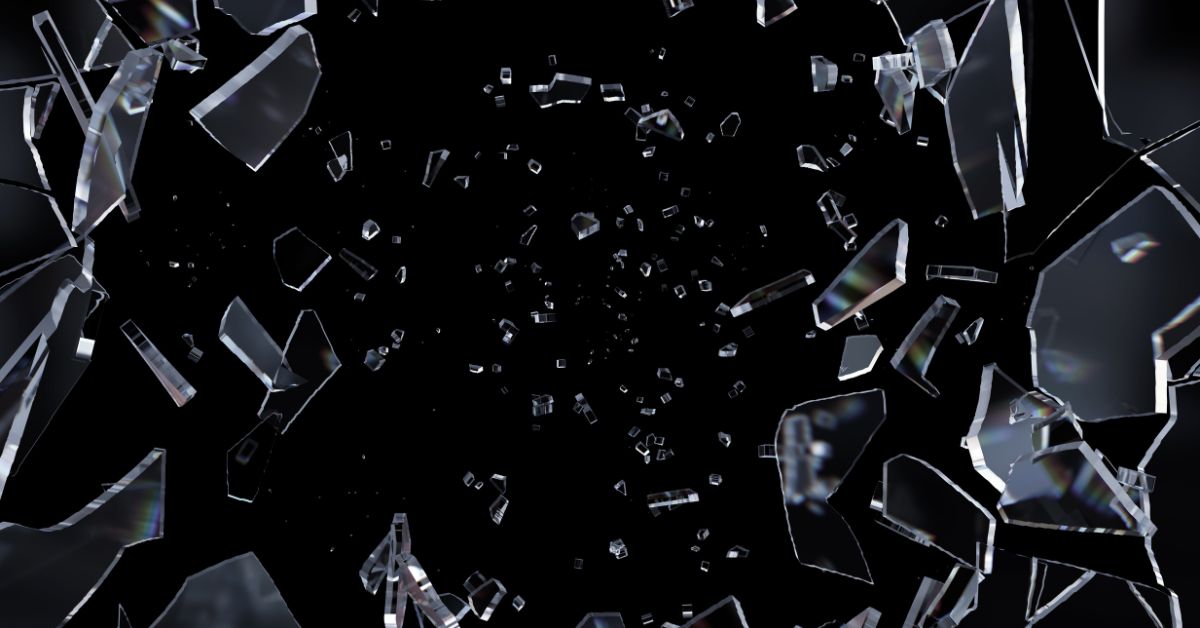
We had the pleasure of speaking with Yasmina Rauber, Secretary General of the Zug Commodity Association, former President of WISTA Switzerland, and Executive Coach at Yas Coaching. Yasmina has built an impressive career in commodities,...
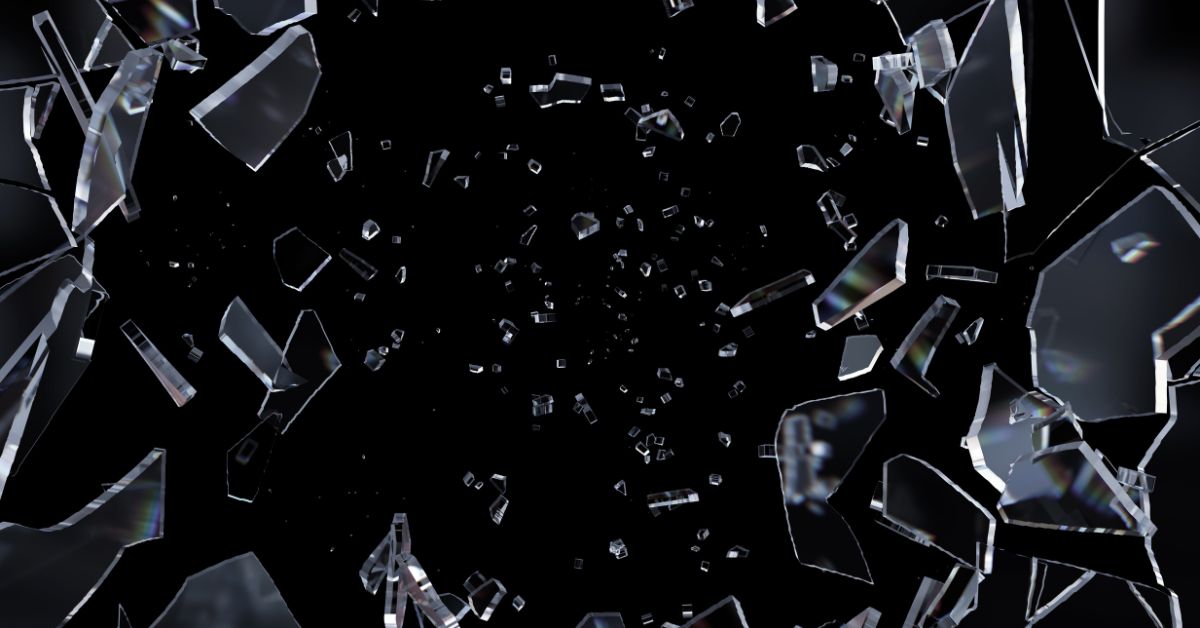
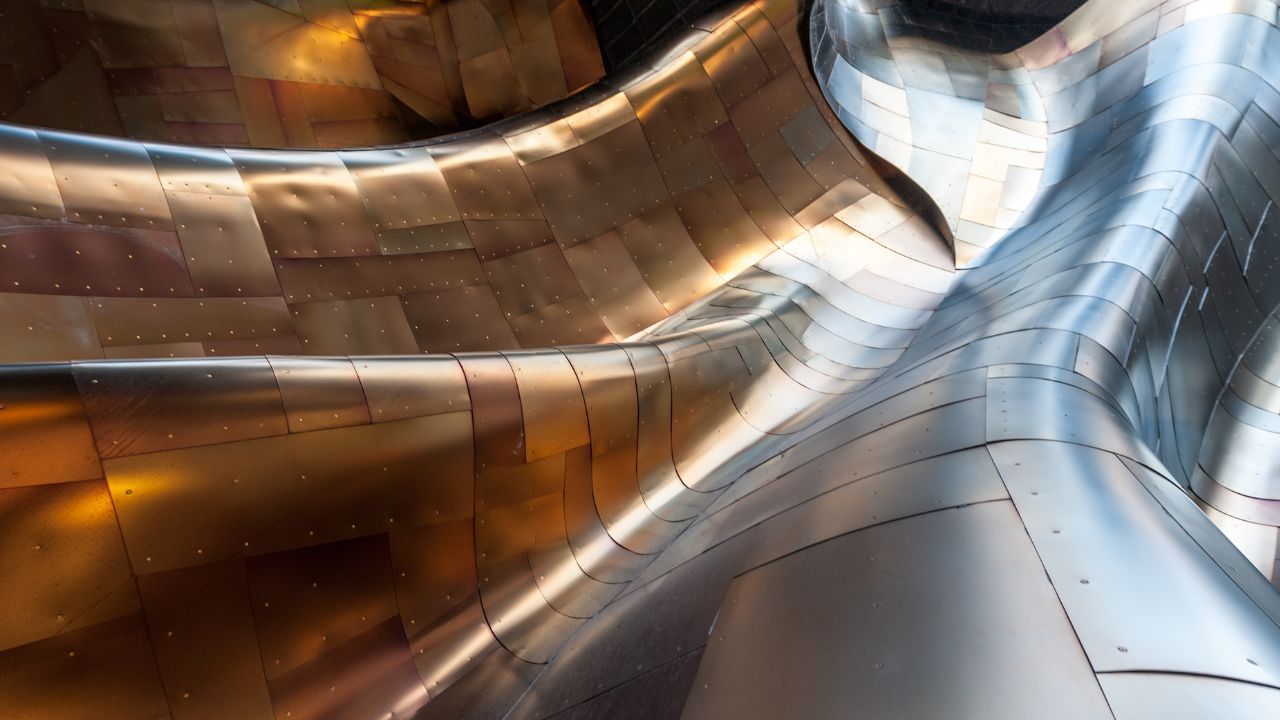
The metals industry has historically been extremely opaque, deliberately so. While some of the largest traders have opened up their books through IPOs, financial reports, and white papers, metals trading remains shrouded in mystery. In...
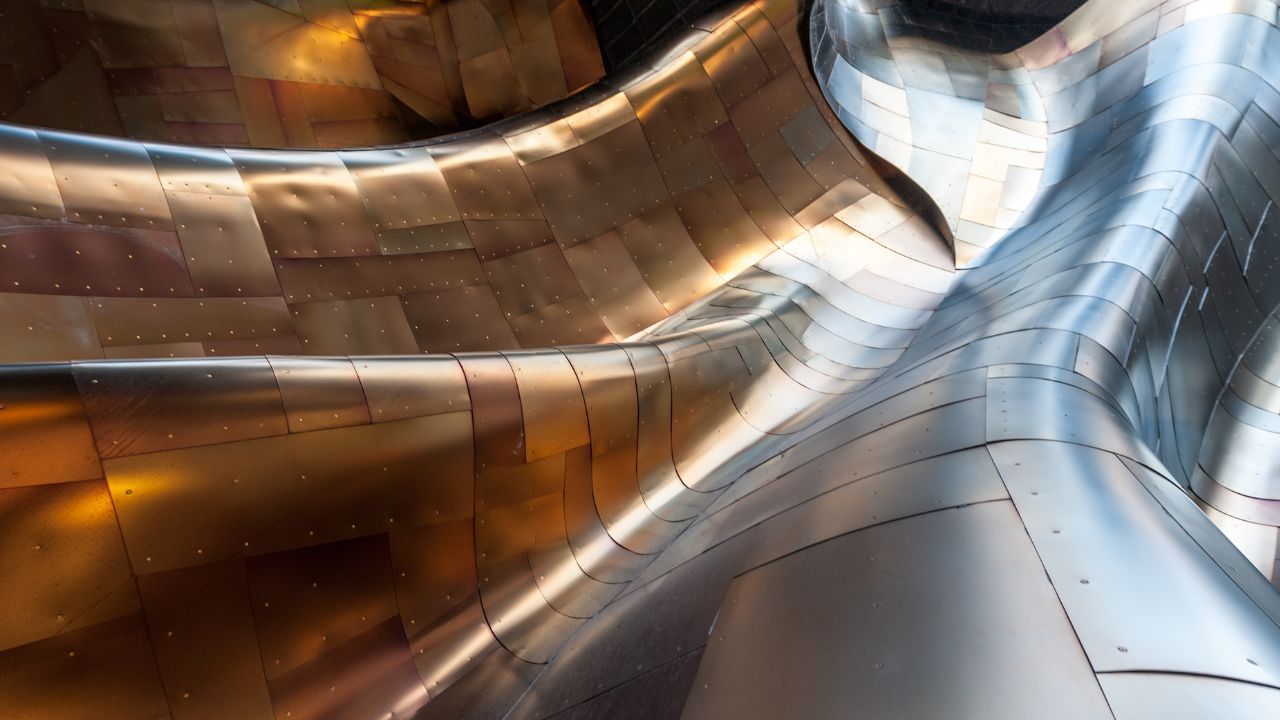